Introduction
Signal Detection Theory (SDT) is a psychological framework developed to explain how individuals differentiate between important stimuli (signals) and irrelevant background noise in their environment. Initially conceptualized during World War II to improve the detection of radar signals, SDT has since found widespread applications in various fields such as psychology, medicine, security, and communications. It is particularly useful in situations where decision-making occurs under conditions of uncertainty and ambiguity.
At its core, SDT recognizes that the detection of a signal is not merely a passive sensory event but involves an active decision-making process. In any given situation, signals are often obscured by noise—irrelevant or distracting stimuli that make it challenging to identify the target. For example, a lifeguard must detect a drowning swimmer (signal) amid the splashing and noise of other swimmers (noise), while a doctor may need to identify a disease marker on a medical scan (signal) amid the natural variations in biological tissues (noise).
SDT provides a structured way to analyze this decision-making process by considering two key components: sensitivity and decision criterion.
Sensitivity refers to the observer’s ability to distinguish between signal and noise, often determined by the strength of the signal relative to the noise and the observer’s perceptual abilities.
Decision criterion, on the other hand, refers to the threshold or standard an observer uses to decide whether the signal is present. An observer can adopt a more liberal criterion, leading to more detections but also more false alarms, or a more conservative criterion, leading to fewer false alarms but potentially more missed signals.
This framework allows researchers to analyze the performance of human observers or systems in detecting signals, taking into account the inevitable trade-offs between correctly identifying signals (hits) and avoiding false alarms. SDT provides valuable insights into how we perceive the world and make critical decisions based on incomplete or ambiguous information, enhancing our understanding of attention, perception, and decision-making in noisy environments.
Basic Concepts of Signal Detection Theory
SDT revolves around how we perceive and detect signals in noisy environments. A signal is any stimulus that we are trying to detect, and noise represents all other irrelevant stimuli or background information that might interfere with that detection. The theory provides insight into how individuals differentiate between significant and insignificant stimuli, even when faced with uncertainty.
The Four Possible Outcomes of Signal Detection
When attempting to detect a target stimulus, there are four possible outcomes, depending on whether the signal is present or absent and whether the observer detects or fails to detect it. These outcomes can be classified into the following categories-
- Hits (True Positives)- The observer correctly identifies the presence of a target signal. For example, a lifeguard correctly spots someone drowning in a crowded pool.
- False Alarms (False Positives)- The observer mistakenly identifies the presence of a target signal when none exists. For example, a lifeguard falsely believes someone is drowning when the person is simply swimming.
- Misses (False Negatives)- The observer fails to detect the presence of a target signal. For instance, a lifeguard fails to notice a person drowning.
- Correct Rejections (True Negatives)- The observer correctly identifies that no target signal is present. For example, a lifeguard correctly recognizes that there is no one in distress in the pool.

Signal Detection Curve
These outcomes reflect the balance between sensitivity to detecting a signal and the observer’s threshold or criterion for declaring something as a signal.
Receiver Operating Characteristic or ROC Curve
In signal detection theory (SDT), one powerful tool used to illustrate the trade-offs between hits and false alarms is the Receiver Operating Characteristic (ROC) curve. The ROC curve is a graphical representation that plots the true positive rate (or sensitivity) against the false positive rate (or 1 – specificity) across various threshold settings. It allows us to visualize how well an observer (or system) can discriminate between signal and noise under different criteria and how changes in the decision threshold affect the balance between hits and false alarms.
The Receiver Operating Characteristic or ROC curve plots the true positive rate or hit (TPR) on the y-axis and the false positive rate or false alarm (FPR) on the x-axis. Each point on the curve corresponds to a specific decision threshold or criterion that the observer uses to declare the presence of a signal. The curve shows the relationship between the observer’s ability to detect true signals (hits) and their susceptibility to false alarms at different decision thresholds.
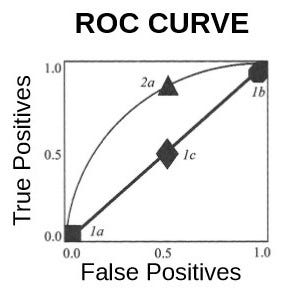
ROC Curve
The curve starts at the origin (0,0), where no signals are detected and no false alarms are made. It then progresses towards the top-right corner (1,1), where all signals and noises are detected as signals (hits and false alarms are maximized). Ideally, the ROC curve would rise steeply towards the top-left corner (0,1), indicating high sensitivity with few false alarms.
Important Factors in Signal Detection Theory
- Decision-Making and Criteria Shifts
SDT also explores how decision-making processes influence the detection of signals. Detection decisions are influenced not only by the observer’s sensitivity to the signal but also by the criteria they set for declaring something a signal. Sensitivity refers to how well the observer can distinguish the signal from the noise, while criteria represent the threshold or standard the observer uses to decide whether a signal is present.
For example, in the case of lifeguards, the consequences of a miss are so severe that they often lower their criteria, accepting more false alarms in the process. This trade-off means that lifeguards are more likely to react to potential signs of danger, even if it results in unnecessary interventions.
In contrast, if the consequences of false alarms are more significant than misses, the observer may raise their criteria. For example, an airport screener may become more conservative in detecting potential threats if too many false alarms (e.g., pulling aside innocent passengers for additional screening) lead to increased wait times and passenger complaints.
- Sensitivity and Bias
Sensitivity (also known as d’) is a measure of how well an observer can distinguish a signal from noise. It reflects the true ability of the observer to detect a stimulus amidst distraction. High sensitivity means that the observer is proficient at separating signal from noise, resulting in more hits and fewer misses.
Bias (also called response criterion or β) refers to the observer’s tendency to Favor one type of response over another. An observer with a liberal bias is more likely to declare that a signal is present, even if it leads to more false alarms. Conversely, an observer with a conservative bias will be more cautious, leading to fewer false alarms but more misses.
In the medical example, a doctor with a liberal bias may err on the side of caution by ordering more tests and making more diagnoses, even if many turn out to be unnecessary. In contrast, a doctor with a conservative bias may be more reluctant to diagnose illnesses, potentially missing some cases but avoiding unnecessary procedures for healthy patients.
Signal Detection in Real-World Contexts
SDT has wide-ranging applications, from medical diagnosis to security screenings and beyond. It is particularly useful in environments where accurate signal detection is crucial and where both misses and false alarms can have serious consequences.
Case Study 1: Lifeguards
Imagine a lifeguard tasked with overseeing a crowded pool. The lifeguard must remain vigilant to detect any signs of drowning, which is often challenging since distress signals can be subtle or obscured by the commotion of others in the water. Lifeguards must rely on signal detection to differentiate between actual emergencies and normal swimming behavior. Their goal is to maximize hits while minimizing false alarms and misses. However, because the stakes are so high—a miss could result in someone’s death—lifeguards are likely to set their detection criteria low, accepting more false alarms in exchange for more hits.
Case Study 2: Medical Diagnosis
Signal detection theory is also vital in medical contexts, especially when doctors use diagnostic tools to detect illnesses. For example, when examining an X-ray or an MRI scan, a doctor must decide whether certain abnormalities suggest the presence of a disease (e.g., cancer). The decision to diagnose or not diagnose an illness hinge on balancing the risk of misses (failing to detect a disease) and false alarms (incorrectly diagnosing a disease). Highly sensitive tests may generate more hits but can also lead to more false alarms, meaning that patients may undergo unnecessary further testing or treatment.
The Signal-Detection Matrix
The signal-detection matrix visually represents the four possible outcomes—hits, misses, false alarms, and correct rejections. This matrix highlights the trade-offs inherent in signal detection, particularly when uncertainty is present. In settings like airport security, where the stakes are high, SDT helps balance sensitivity and specificity in detecting potential threats like weapons or explosives.

Signal Detection Matrix
Applications of Signal Detection Theory
Signal detection theory has many real-world applications, ranging from security screening and medical diagnostics to education, sports, and even marketing.
- Security Screening
SDT is often applied in contexts where vigilance and attention are critical to safety, such as airport security. Airport screeners must scan passengers’ luggage for prohibited items like weapons or explosives. This task requires them to constantly detect signals (e.g., a gun or knife) within a noisy background (e.g., electronics, clothing, personal items).
One of the challenges in airport security is balancing sensitivity to potential threats with minimizing false alarms, which can lead to unnecessary delays and frustrations for passengers. After the 9/11 attacks, airport security screening protocols became stricter, resulting in more false alarms as screeners pulled aside travelers for additional checks. Over time, these procedures were refined to reduce false alarms by using advanced screening technologies and implementing more sophisticated passenger profiling methods.
In medicine, SDT helps clinicians make accurate diagnoses. Consider a screening test for cancer. A doctor must decide whether the test results indicate the presence of cancer or whether they are simply noise. This decision is based on the doctor’s sensitivity to detecting abnormal cells and their criteria for declaring the presence of cancer. A more sensitive test may result in more hits (correct diagnoses), but it may also lead to more false alarms (incorrect diagnoses). On the other hand, if the criteria for diagnosis are too high, the doctor may miss cases of cancer that should have been treated.
- Education and Assessment
SDT is also applied in education, particularly in testing and assessment. When grading exams or evaluating student performance, educators must decide whether a student has met certain learning objectives (the signal) or not (the noise). The challenge lies in setting the right criteria for passing or failing, particularly in subjective assessments where the distinction between success and failure may not be clear-cut.
- Sports
In sports, SDT plays a role in performance and decision-making. Athletes and coaches must quickly identify important signals (e.g., a ball coming toward them) amidst distractions (e.g., noise from the crowd, movement from other players). For example, a soccer goalkeeper must decide whether an incoming shot is going toward the goal (a hit) or not (a false alarm), while also considering the risks of making incorrect judgments.
- Marketing
In marketing, companies use SDT principles to design advertisements and campaigns that stand out from the noise of competing messages. Marketers aim to maximize hits by creating advertisements that effectively capture consumers’ attention while minimizing false alarms (i.e., consumers being misled or annoyed by irrelevant messages).
The Role of Attention, Perception, and Memory in Signal Detection
SDT can be understood in the context of three key cognitive processes: attention, perception, and memory.
- Attention– Attention refers to the process of focusing on specific stimuli while ignoring others. In the context of SDT, paying enough attention to perceive objects or signals that are present is critical. Lifeguards, airport screeners, and doctors must maintain high levels of attention to ensure they detect important signals. In high-pressure environments, such as air traffic control or emergency response, sustained attention, also known as vigilance, is crucial for accurate signal detection.
- Perception– Perception involves the interpretation of sensory information. In signal detection, perception is necessary for recognizing faint or subtle signals that might be difficult to detect due to noise. For instance, a doctor might perceive faint abnormalities in a medical scan that are barely noticeable. In other cases, individuals might need to detect signals beyond their perceptual range, such as hearing a high-pitched tone or noticing a brief flash of light.
- Memory– Memory plays a role in SDT when individuals must recall whether they have been exposed to a particular stimulus before. For example, during a memory test, individuals may be asked to indicate whether they recognize specific words from a list. Signal detection is used to determine whether the individuals can accurately distinguish between words they have seen before (hits) and new words that are similar but have not been presented (false alarms).
Evolving Signal Detection Systems
Signal detection systems are constantly evolving in response to the changing nature of the environments in which they operate. In many fields, such as security and healthcare, technological advancements are helping to improve sensitivity and reduce false alarms.
For instance, modern airport screening technologies now use advanced imaging systems that provide more accurate representations of objects inside luggage, reducing the likelihood of false alarms. Similarly, in healthcare, machine learning algorithms are being developed to assist doctors in detecting diseases with greater accuracy, reducing both misses and false alarms.
Conclusion
Signal Detection Theory (SDT) provides a robust framework for understanding how people make decisions in complex, uncertain environments, offering valuable insights into how we perceive, attend to, and respond to stimuli amidst noise. From its origins in radar and communications during World War II to its contemporary applications in fields like psychology, medicine, and security, SDT has proven its versatility in explaining how decisions are made when there is no clear separation between signal and noise.
One of the major contributions of SDT is its ability to dissect the process of decision-making into measurable components—namely, sensitivity and decision criterion. Sensitivity refers to the inherent ability of the observer or system to distinguish a signal from noise. This ability can be influenced by various factors, including the clarity or strength of the signal, the amount of noise present, and the observer’s perceptual abilities. For example, in a medical setting, a radiologist’s sensitivity might depend on their training and experience, as well as the quality of the imaging tools they use.
Decision criterion, on the other hand, represents the observer’s strategy or threshold for determining whether a signal is present. This criterion is adjustable and reflects the individual’s or system’s willingness to trade-off between hits (correct signal detections) and false alarms (incorrectly identifying noise as a signal). For example, a lifeguard monitoring a crowded pool may lower their decision threshold to maximize the detection of swimmers in distress, knowing that this could lead to an increase in false alarms but would reduce the risk of missing an actual emergency.
SDT’s ability to quantify these trade-offs has made it particularly useful in critical fields such as medicine and security, where the cost of a missed signal can be extremely high. In medical diagnosis, for example, the consequences of a miss could mean failing to detect a life-threatening condition, while a false alarm could lead to unnecessary treatments and patient anxiety. Similarly, in airport security, a missed detection could result in a catastrophic event, while a false alarm may inconvenience passengers and overwhelm security personnel.
SDT provides a framework for making these critical decisions more manageable by allowing decision-makers to set their criteria based on the specific risks and costs associated with misses and false alarms. In medical settings, doctors can use SDT to evaluate the effectiveness of diagnostic tests by balancing sensitivity (correct diagnoses) with specificity (avoiding false positives). Similarly, in military or security contexts, personnel can use SDT to fine-tune their surveillance systems and protocols, ensuring that they catch potential threats without being overwhelmed by false alarms.
Beyond its practical applications, SDT also offers profound insights into the cognitive processes underlying decision-making. The theory bridges the gap between sensory input and cognitive interpretation, recognizing that perception is not simply a passive reception of external stimuli but an active process influenced by biases, prior experiences, and the current environment. This perspective has had significant implications for psychological research, particularly in understanding how attention, perception, and memory interact to influence our decisions.
For instance, SDT has been instrumental in understanding how attention operates in noisy environments, such as air traffic control or high-pressure medical situations. In these scenarios, the observer must focus attention on relevant signals while ignoring distractions. SDT helps clarify how different attentional strategies might affect performance, providing insight into how training and experience can improve decision-making accuracy.
In memory research, SDT helps differentiate between genuine memories and false recognitions. By using the SDT framework, researchers can measure how well people remember events and how often they falsely recall details, contributing to a deeper understanding of how memory works and how it can be manipulated or distorted.
One of the key strengths of SDT is its flexibility and adaptability. Although it began as a tool for analysing radar detection, it has been applied successfully in numerous fields, from marketing to cognitive psychology, and from medical diagnostics to national security. The theory’s fundamental principles—balancing sensitivity and decision-making thresholds—are universally relevant, making it an essential tool for anyone who needs to make decisions under uncertainty.
Despite its broad applicability, SDT is not without limitations. For example, it assumes that the decision-making process is based on a single signal embedded in noise, which may not always be the case in real-world settings where multiple signals or different types of noise are present. Furthermore, the theory assumes that decision thresholds remain consistent across time, yet in practice, these thresholds may shift dynamically based on feedback, changing environments, or cognitive fatigue.
Nevertheless, the enduring appeal of SDT lies in its ability to simplify complex decision-making processes while still offering a rich understanding of the cognitive mechanisms at play. Its ability to quantify and model human perception and decision-making has led to improvements in everything from medical diagnostics to military strategy, and from consumer behavior to everyday decision-making.
References
Macmillan, N. A. (2002). Signal detection theory. Stevens’ handbook of experimental psychology: Methodology in experimental psychology, 4, 43-90.
McNicol, D. (2005). A primer of signal detection theory. Psychology Press.
Sternberg, R. J., & Sternberg, K. (2006). Cognitive psychology (p. 178). Belmont, CA: Thomson/Wadsworth.
Subscribe to Careershodh
Get the latest updates and insights.
Join 15,002 other subscribers!
Niwlikar, B. A. (2024, September 22). Signal Detection Theory- Discover the 2 Insightful Factors that Influence Decision Making.. Careershodh. https://www.careershodh.com/signal-detection-theory/